The energy crisis is hitting the European manufacturing industry. Hard. Till a few months ago, labor cost was the largest price driver for base materials and semi products on the Old Continent. Since a few months however the balance has shifted: energy costs represent the biggest contributor to that overall cost. As a result multiple production sites have been shut down, or didn’t start up again after the summer maintenance break. Today some are even afraid that many of the closures will turn into permanent shutdowns, further reducing the economical importance of this sector and the associated jobs as well as independence of other regions.
To limit the impact, of course a main focus should lie on keeping energy costs below certain limits. Next to these external factors, companies should also focus inwards: make sure the energy required per component or unit of mass produced can be kept a minimum. Energy needs depend on process design and have a ‘minimum’, as thermodynamics cannot be neglected. But even in an existing plant there’s multiple additional optimizations that can be implemented. Many of these can be identified by the principle that measuring and monitoring allow to detect issues (like temporarily excessive consumption) and lead to an improved understanding (offering options to fundamentally improve operations).
The present article will highlight a number of approaches, ranging from more simple and relatively straightforward in implementation (based on simple threshold values: detection), towards more advanced solutions (based on more complex automated analysis, including prediction).
- Monitor energy consumption. Install a number of smart meters or current coils in the site or plant. For each meter: list the assets for which the current/powers passes through it. Log power consumption on a daily or hourly basis and identify the biggest contributors (energy consumption per day or per week). Study how they work and find possible savings in an engineering way: from the analysis determine what can be done or changed. By continued monitoring the impact of the intervention can be quantified.
- A next level can be reached when monitoring with higher resolution, in space and time: install power measurement hardware on an individual machine or installation and monitor power consumption on a 10 or 1-minute basis. As a result of this increased granularity the actual energy consumption of the specific unit can be monitored continuously and if this turns out to be exceeding the usual ‘per minute’ of ‘per 10 minutes’ consumption, deviations can be detected in real-time, and not at the end of the month, a typical reporting period used in organizations. The information related to the asset affected can in turn be shared with engineering or maintenance, with as a goal to correct or improve the situation.
- A more advanced approach involves really stepping into the process. This requires having the hardware to monitor energy consumption, for an individual installation, per minute or per 10 minutes. Next to this also the data related to the production volume: mass per minute or items per hour need to be sourced. When done on daily basis (how much did we produce today + how much energy was consumed), a rough estimate can be generated. An alternative approach is however related to implementing some smart algorithms. These allow deriving, from the power signal, those periods in the day when the machine or line was running in a normal regime, without interruptions: on-line classification. From the second data source, using these time stamps, the exact number of items or mass of product created is derived. Combining both the actual energy consumption per unit can be calculated, as an on-line parameter, and tracked. By dividing the total energy consumed when ‘in regime’ by the total energy consumed by the machine, the actual ‘useful energy consumption’ can be determined. Knowing the total energy consumption this also provides one with the amount of energy consumed under sub-optimal producing conditions, e.g. when the line wasn’t in regime, when the products made were not up to spec (so had to be discarded)…: quantification. Identifying (also automatically, in the same data platform) the top 3 causes of these energy losses represents a next potential pathway to energy cost reductions.
In reality, for energy-intensive processes, it turns out that indeed a lot of electricity, steam, gas… is lost during so-called transitions: at startup, when stopping, or when operating in a non-optimal way (producing material that is not within required specifications). Ovens have to be heated up again to the appropriate process temperature, machines that have to produce for several tens of minutes before the optimal set points are reached… All the associated energy can be saved if these transitions can be avoided.
This can also be achieved through monitoring, though in a slightly more advanced way. If upcoming damage to a machine, line or installation can be predicted, the only stops experienced will be prepared and planned. A first outcome is that the overall number of stops will be reduced. A second outcome is that proper shutdown procedures can be followed and maximal repairs and optimizations can be performed during shutdown. This again leading to further reduction in future unanticipated stops.
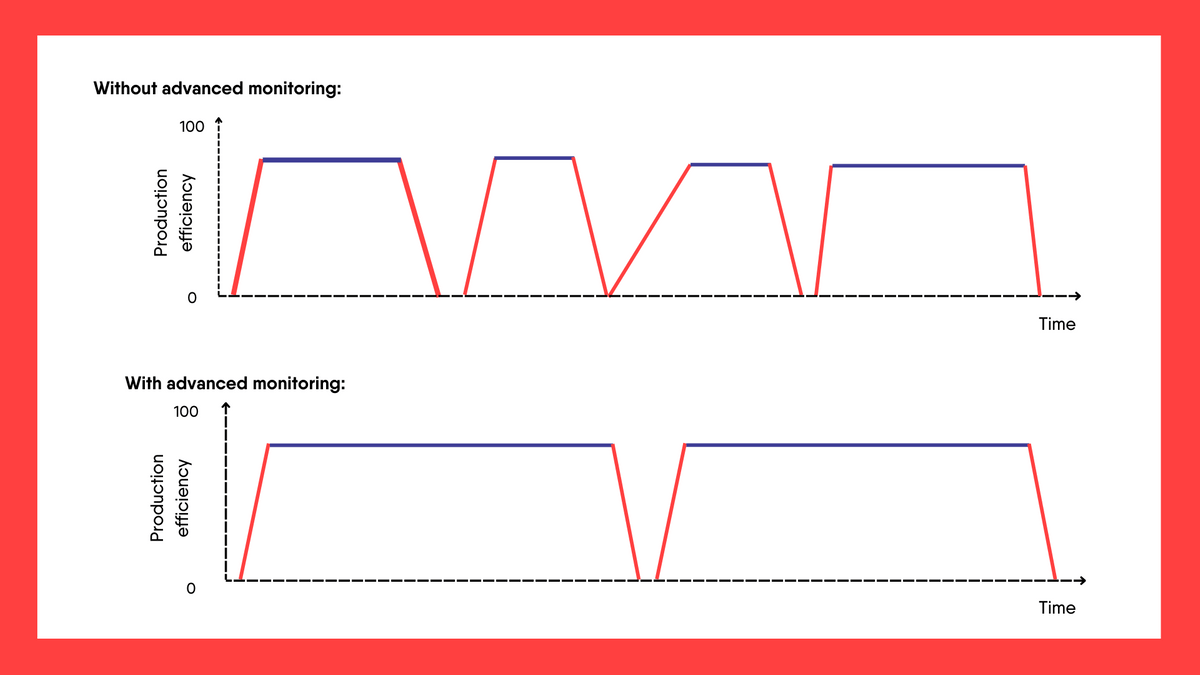
Next to predicting upcoming issues, also reasons of failure or accelerated wear can be identified. By looking at these in more detail, a next level can be obtained: preventing similar damage in the future, hence also the associated costs for energy, materials and working hours. To achieve this, a more complete approach is required: monitoring energy consumption ideally at 1 Hz or higher, ingest data about production volumes, but also by including additional physical measurements (often at higher data rates) such as vibrations, temperatures, inclinations… The algorithms applied will initially again rely on a classification layer, but in further stages use the physics associated to the machine type and specific AI-based models to discriminate normal from abnormal operations, detect trends and count the number of shocks and hiccups in the process.
In short: by carefully quantifying energy consumption in absolute numbers (per production volume) and by reducing the number of unforeseen stops the total energy consumption if a site can be drastically reduced. In a rough estimate: reducing the number of days of unforeseen standstill from 6 to 3 a year has a total energy savings potential of 0,8% of the annual consumption. Worth considering as an investment?